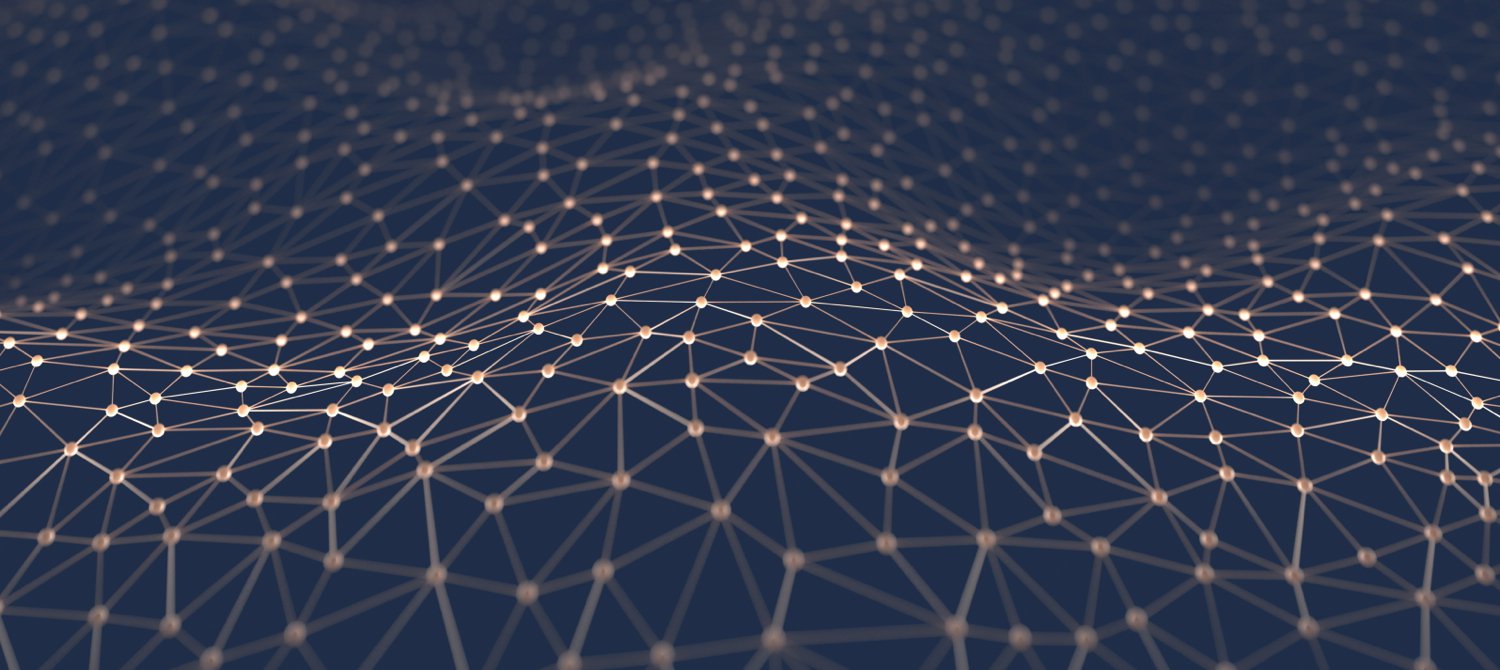
ORCAA’s dual mission is to help define accountability for algorithms, and to keep people safe from harmful consequences of AI and automated systems. Whether it’s a hiring algorithm, healthcare AI, predictive scoring system, or generative AI platform, we are here to think about how it could fail, for whom, and what you can do to monitor and mitigate these risks. We are actively developing frameworks and governance approaches to help companies and organizations use algorithms and AI safely – confirming these technologies perform as intended and operate within sensible guardrails. We help our clients realize the transformative benefits of AI, while avoiding discrimination, bias, and other problems.
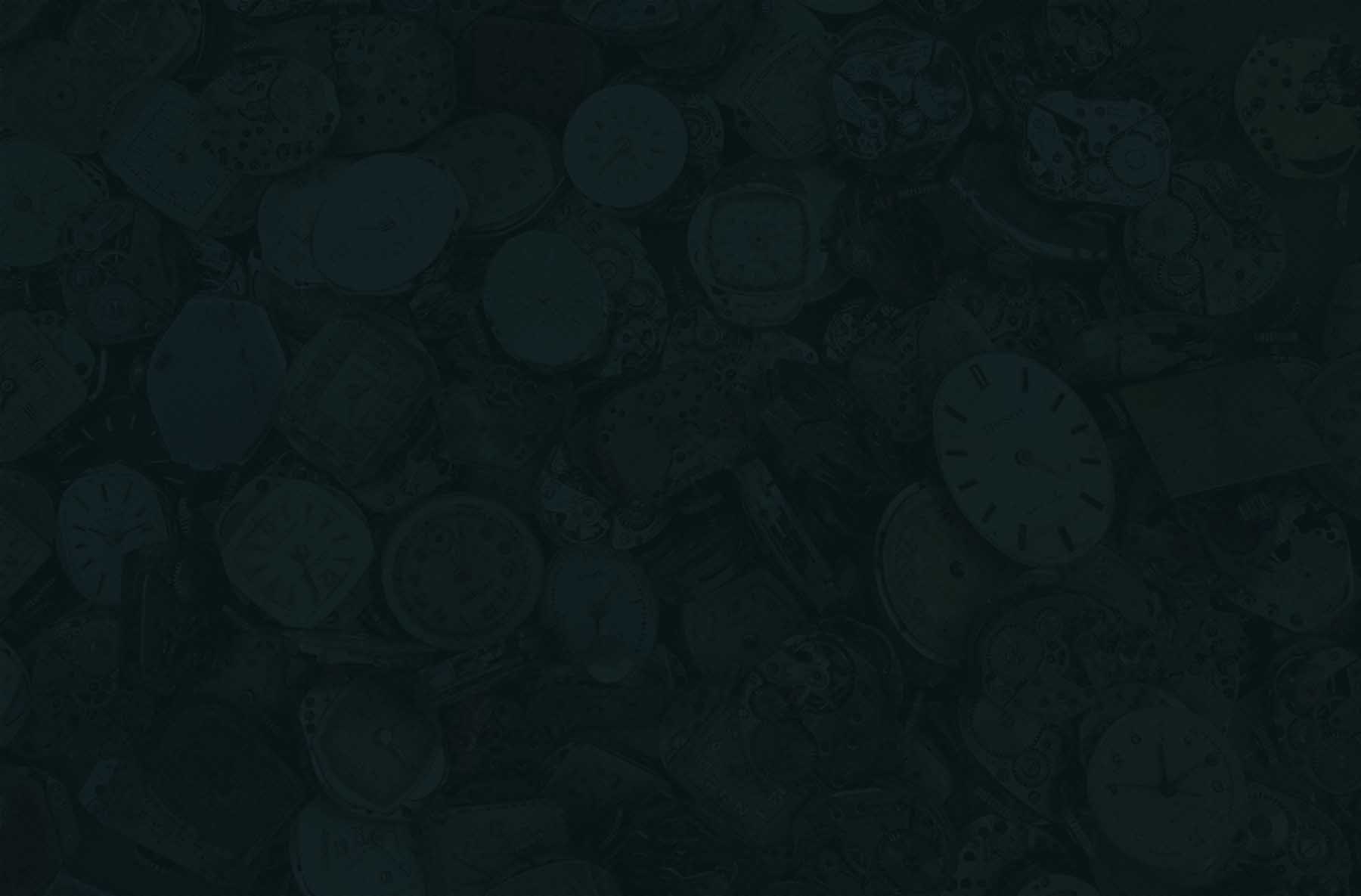
Services
Algorithmic Audit
A comprehensive assessment of risks associated with a specific use case of an algorithmic system. We audit systems of all kinds, including generative AI, automated decision systems, predictive models, and facial recognition.
Uses our Ethical Matrix framework
Identifies high-priority issues around fairness and performance
Delivers recommendations for measuring and mitigating risks
Outputs / Deliverables: Algorithmic Audit Report
Pilot: Quantitative testing for regulatory compliance and more
Analysis to measure bias in algorithmic systems, using data from live deployments or test data.
Proprietary, patent-pending cloud based analysis platform
Incorporates inference methodologies, so we can measure gender and race/ethnicity bias even if you do not possess this data
Double-firewall privacy protection: we never see personally-identifying information; client never sees individual inferences
Outputs / Deliverables: Bias Audit Report (e.g. for NYC Local Law 144); custom analysis reports
AI Governance + Risk Management Consultation
We help build core infrastructure to help you use AI responsibly.
Develop an organization-level approach to measuring and managing the risks of deployed AI systems, especially with regard to bias/discrimination
Review and recommend governance policies, processes, and structures
Assistance with procurement of AI technologies from vendors and other 3rd parties
Outputs / Deliverables: Documentation of risk management structures; diligence reports on prospective vendors
Cockpit Design
Running a deployed AI system is like piloting a plane: to fly safely, you need critical information in real time. To make an effective cockpit, you must understand what can go wrong in flight, and include dials/gauges that monitor for those risks. We help build cockpits for your AI systems.
Identify and/or construct metrics that address key risks
Calibrate thresholds and develop mitigation tactics
Can work with existing analytics platforms or assist with your custom implementation
Education + Training
We teach people how to think critically about AI and algorithmic systems, how to identify blind spots, and about the craft of auditing.
Workshops
Bespoke training for audit and risk management teams
Industries + Clients
Our partners range from silicon valley to the DMV, including private and public companies of all sizes and public agencies, in the US and internationally. We have a special interest in algorithms being used for hiring, insurance, credit, education, and healthcare – because we think algorithms in these sectors have major impacts on people’s lives, and need to be monitored closely.
Past and current clients include:
Explainable Fairness
When is an algorithm fair?
We propose the Explainable Fairness framework: a process to define and measure when an algorithm is complying with an anti-discrimination law. The central question is, Does the algorithm treat certain groups of people differently than others? The framework has three steps: choose a protected class, choose an outcome of interest, and measure and explain differences.
Example from hiring algorithms
Step 1: Identify protected stakeholder groups. Fair hiring rules prohibit discrimination by employers according to gender, race, national origin, and disability, among other protected classes. So all these could be considered specific groups for whom fairness needs to be verified.
Step 2: Identify outcomes of interest. In hiring, being offered a job is an obvious topline outcome. Other outcomes could also be considered employment decisions: for instance, whether a candidate gets screened out at the resume stage, or whether they are invited to interview, or who applied in the first place, which might reflect bias in recruitment.
Step 3: Measure and Explain Loop. Measure the outcomes of interest for different categories of the protected class. For example, are fewer women getting interviews? If so, is there a legitimate factor that explains that difference? For example, are men who apply more likely to have a relevant credential or more years of experience? If so, account for those legitimate factors and remeasure the outcomes. If you end up with unexplained large differences, you have a problem.
The process can be applied more generally, and looks like this:
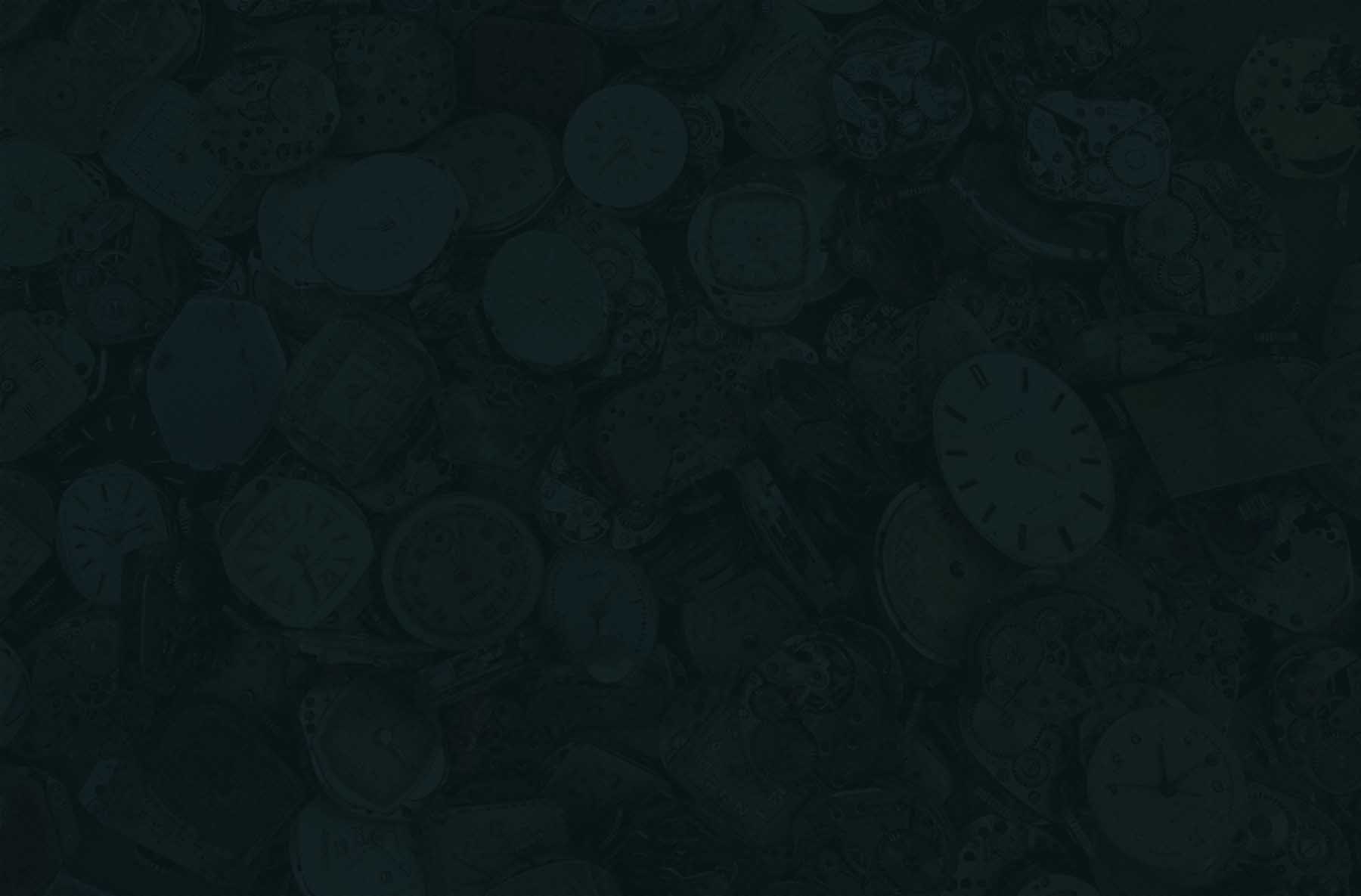
Bias Audits for NYC Local Law 144
The new NYC law Int 1894-2020 (“Local Law 144”) requires annual bias audits of all automatic hiring decision tools used to hire candidates in or from NYC.
We offer a Bias Audit service to help companies comply with this law. We use our Pilot analysis platform to conduct disparate-impact-style and other analyses on real-world data arising from a specific use of a given decision tool. To show what we mean, here is a mock Bias Audit report for a fictitious company NewCo, which is using fictitious ToolX in its hiring process.
We can conduct a Bias Audit whether you are a vendor building hiring tools to be used by others, or a company using an AI tool in your own hiring process. If you already have race/ethnicity or gender information about candidates, we can use it in the audit; if you do not, we offer inference methods to model this information.
Please contact us to learn more about getting a Bias Audit.
HTI-1 Final Rule Reporting
How We Can Help
ORCAA and DIHI are proud to offer HTI-1 compliance reporting research and support services. ONC’s December 2023 HTI-1 Final Rule requires health IT developers to conduct more diligence than ever before about their Predictive Decision Support Interventions (Predictive DSIs) -- and provide more detailed reporting. We offer end-to-end support to navigate these requirements, including:
Developing use-case-specific metrics and processes for ensuring fairness and validating performance of Predictive DSIs
Curating data for and conducting external research on the validity and fairness of Predictive DSIs, and
Preparing and reviewing compliance reports.
Whether you are starting from scratch to meet HTI-1 requirements or have your compliance reports drafted and simply want an independent expert review, we can help. Please see below our research and service offering details to meet each section of the requirements:
-
image01
About the Partnership
This partnership represents a unique combination of expertise. ORCAA is a leading algorithmic auditing consultancy, focused on developing and applying standards for algorithmic systems. Our experience -- with diverse clients including private firms, regulatory agencies, and AGs; and across industries with different regulatory regimes -- gives us a broad perspective on how to demonstrate that algorithmic systems are safe and fair. ORCAA is an inaugural member of the US AI Safety Institute Consortium. Duke Institute for Health Innovation (DIHI) brings over ten years of real-world experience translating ideas into sustainable health innovations, including the sourcing, design, development, and implementation of more than 20 AI-based solutions into clinical care. DIHI is also the coordinating center for Health AI Partnership, a multi-stakeholder collaborative to advance responsible and equitable use of AI in healthcare.
Principles
Context Matters
An algorithm isn’t good or bad per se – it is just a tool. Who is using it, and to what end? What are the consequences? We go beyond traditional accuracy metrics to ask how the algorithm is used to make decisions, and who is affected.
Putting the science into data science
Graphs and statistical tests can be useful – if they answer a meaningful question. We translate specific ideas and concerns about fairness into statements we can test empirically. Then we design experiments to say whether those standards are being met.
AI Ethics cannot be automated
There cannot be a universal algorithmic auditing checklist or a fully automated review process – context is too important. An audit for a specific purpose may be repeatable, but human discussion and judgment will always be essential. We will tailor a tool to your needs, not repurpose what worked in another setting.
Contact
We are ORCAA
Cathy O’Neil
CEO
Cathy has been an independent data science consultant since 2012 and has worked for clients including the Illinois Attorney General’s Office and Consumer Reports. She wrote the book Doing Data Science in 2013 and Weapons of Math Destruction: How Big Data Increases Inequality And Threatens Democracy, released in September 2016.
Tom Adams
COO and General Counsel
Thomas Adams has over twenty-five years of business and legal experience. He has represented banks, companies and individuals on corporate, securities and business law matters. He also provided strategic advice, litigation support and expert witness testimony on issues relating to the financial crisis. Mr. Adams is an expert in creating solutions and solving problems for complex financial and corporate transactions and has provided strategic advice and analysis to banks, insurance companies, private equity companies, hedge funds and a variety of other companies. He graduated from Fordham Law School in 1989 and Colgate University in 1986. He is admitted to practice in New York.
Jacob Appel
Chief Strategist
Jake is ORCAA’s Chief Strategist. He conducts algorithmic audits, and specializes in designing tests and analyses to assess the performance of algorithms and their impacts on stakeholders. Before joining ORCAA he worked with the Behavioral Insights Team, where he advised state and local governments on incorporating behavioral science “nudges” into citizen-facing policies and programs, and testing them with randomized experiments. Jake received a BS in mathematics from Columbia University and an MPA from Princeton School of Public and International Affairs. He coauthored two books: More Than Good Intentions: How a new economics is helping to solve global poverty, and Failing in the Field: What we can learn when field research goes wrong.
Meredith Broussard
Affiliate
Data journalist Meredith Broussard is an associate professor at the Arthur L. Carter Journalism Institute of New York University, research director at the NYU Alliance for Public Interest Technology, and the author of “Artificial Unintelligence: How Computers Misunderstand the World.” Her academic research focuses on artificial intelligence in investigative reporting and ethical AI, with a particular interest in using data analysis for social good. She appeared in the 2020 documentary Coded Bias, an official selection of the Sundance Film Festival that was nominated for an NAACP Image Award. She is an affiliate faculty member at the Moore Sloan Data Science Environment at the NYU Center for Data Science, a 2019 Reynolds Journalism Institute Fellow, and her work has been supported by New America, the Institute of Museum & Library Services, and the Tow Center at Columbia Journalism School. A former features editor at the Philadelphia Inquirer, she has also worked as a software developer at AT&T Bell Labs and the MIT Media Lab. Her features and essays have appeared in The Atlantic, The New York Times, Slate, and other outlets.
Şerife (Sherry) Wong
Affiliate
Şerife (Sherry) Wong is an artist and founder of Icarus Salon, an art and research organization exploring the societal implications of emerging technology. She is a researcher at the Berggruen Institute where she focuses on the data economy for the Transformations of the Human program, serves on the board of directors for Digital Peace Now, and is a member of Tech Inquiry. She has been a resident on artificial intelligence at the Rockefeller Foundation Bellagio Center, a jury member at Ars Electronica for the European Commission, and frequently collaborates on AI governance projects with the Center for Advanced Study in the Behavioral Sciences at Stanford. Previously, she created the Impact Residency at Autodesk’s Pier 9 Technology Center where she worked with over 100 leading creative technologists exploring the future of robotics, AR/VR, engineering, computer-aided machining, and machine learning for product development, and worked at the Electronic Frontier Foundation.
Betty O’Neil
Affiliate
Betty O’Neil (really Elizabeth) is a computer scientist specializing in database internals, and is also interested in how computers can be used to make the world a better place. Like her daughter Cathy, she earned a PhD in Mathematics (Applied in her case) at Harvard to get started. She was a professor at the University of Massachusetts Boston for many years, and now is joining ORCAA’s efforts in using data science in socially responsible ways. She is a co-author of a graduate database textbook. As a database internals expert, she has helped implement several important databases, including Microsoft SQL Server (two patents in 2001), and more recently, Stonebraker’s Vertica and VoltDB. She is a lifelong nerd and can program anything.
Deborah Raji
Affiliate
Deborah Raji is an affiliate at ORCAA. She has worked closely with the Algorithmic Justice League initiative, founded by Joy Buolamwini of the MIT Media Lab, on several award-winning projects to highlight cases of bias in facial recognition. She was a mentee in Google AI’s flagship research mentorship cohort, working with their Ethical AI team on various projects to operationalize ethical considerations in ML practice, including the Model Cards documentation project, and SMACTR internal auditing framework. She was also recently a research fellow at the Partnership on AI, working on formalizing documentation practice in Machine Learning through their ABOUT ML initiative, as well as pushing forward benchmarking and model evaluation norms. She is a Mozilla fellow and was recently named as one of MIT Tech Review’s 35 Innovators Under 35. She is currently pursuing a Ph.D in Computer Science at UC Berkeley.
Anna Zink
Affiliate
Anna Zink is a principal researcher at Chicago Booth's Center for Applied AI where she works on their algorithmic bias initiative. Her research is focused on algorithmic fairness applications in health care, including the evaluation of risk adjustment formulas used for health plan payments. Before receiving her PhD in Health Policy from Harvard University, she worked as a data analyst at Acumen, LLC. where, among a small team of analysts, she partnered with the Department of Justice on cases of Medicare fraud, waste, and abuse and helped develop fraud surveillance methods.
Emma Pierson
Affiliate
Emma Pierson is an assistant professor of computer science at the Jacobs Technion-Cornell Institute at Cornell Tech and the Technion, and a computer science field member at Cornell University. She holds a secondary joint appointment as an Assistant Professor of Population Health Sciences at Weill Cornell Medical College. She develops data science and machine learning methods to study inequality and healthcare. Her work has been recognized by best paper, poster, and talk awards, an NSF CAREER award, a Rhodes Scholarship, Hertz Fellowship, Rising Star in EECS, MIT Technology Review 35 Innovators Under 35, and Forbes 30 Under 30 in Science. Her research has been published at venues including ICML, KDD, WWW, Nature, and Nature Medicine, and she has also written for The New York Times, FiveThirtyEight, Wired, and various other publications.
Shamus Khan
Affiliate
Shamus Khan is Willard Thorp professor of sociology and American Studies at Princeton University. He is the author of over 100 articles, books, and essays, including Privilege: The Making of an Adolescent Elite at St. Paul’s School, and Sexual Citizens: Sex, Power, and Assault on Campus (with Jennifer Hirsch), one of NPR’s best books of 2020. He writes regularly in the New York Times and Washington Post. He has been awarded Columbia University’s highest teaching honor, the Presidential Teaching Award (2016), and the Zetterberg Prize from the Upsala University for “the best sociologist under 40” (2018).
Kristopher Velasco
Affiliate
Kristopher Velasco (he/him/his) is an Assistant Professor in the Department of Sociology at Princeton University. Kristopher’s research is driven by one overarching question: how do organizations and institutions facilitate social and cultural change? He addresses this question by focusing on changing understandings of gender and sexuality and the backlash this invites. Kristopher has received awards and grants for his research from the American Sociological Association, American Political Science Association, International Studies Association, Academy of Management, the Ford Foundation, and the National Science Foundation. Kristopher received his B.A. from the University of Kansas and M.A. and Ph.D. from the University of Texas at Austin.